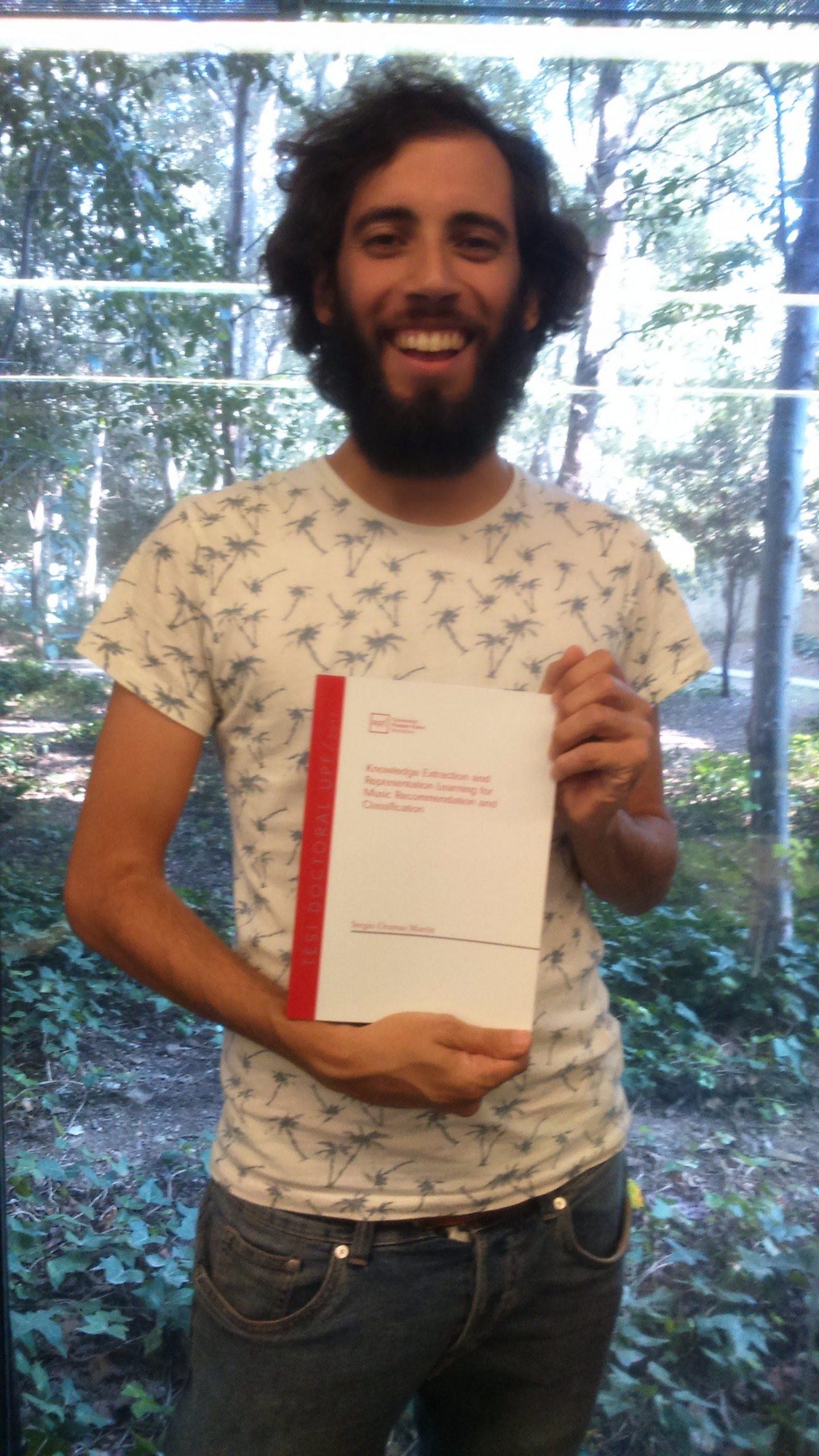
PhD Thesis
Oramas, S. (2017). Knowledge Extraction and Representation Learning for Music Recommendation and Classification. PhD thesis, Universitat Pompeu Fabra, Barcelona, Spain.
Short Abstract
In this thesis, we address the problems of classifying and recommending music present in large collections. We focus on the semantic enrichment of descriptions associated to musical items (e.g., artists biographies, album reviews, metadata), and the exploitation of multimodal data (e.g., text, audio, images). To this end, we first focus on the problem of linking music-related texts with online knowledge repositories and on the automated construction of music knowledge bases. Then, we show how modeling semantic information may impact musicological studies and helps to outperform purely text-based approaches in music similarity, classification, and recommendation. Next, we focus on learning new data representations from multimodal content using deep learning architectures, addressing the problems of cold-start music recommendation and multi-label music genre classification, combining audio, text, and images. We show how the semantic enrichment of texts and the combination of learned data representations improve the performance on both tasks.
Datasets
- ELMD Dataset of ∼13k documents and almost 150k annotated musical entities, which are linked to DBpedia and MusicBrainz. From this corpus, a gold standard dataset of 200 documents with manually annotated entities is also created. http://mtg.upf.edu/download/datasets/elmd
- MARD Large dataset of about 64k albums with customer reviews, acoustic features per track, metadata, and single-label genre annotations. http://mtg.upf.edu/download/datasets/mard
- SAS Two datasets of 188 and 2,336 artist biographies respectively, together with artist similarity ground truth data. http://mtg.upf.edu/download/datasets/semantic-similarity
- KG-Rec Two datasets of tags and text descriptions about musical items, together with user feedback information on those items. A dataset of sounds with ∼21k items and 20k users, and a dataset of songs with ∼8.5k items and ∼5k users. http://mtg.upf.edu/download/datasets/knowledge-graph-rec
- MSD-A Dataset of ∼24k artist biographies linked to the artists present in the Million Song Dataset. http://mtg.upf.edu/download/datasets/msd-a
- MuMu Large dataset of about ∼31k albums, with ∼450k customer reviews, ∼147k audio tracks, cover artworks, and multi-label genre annotations. https://www.upf.edu/web/mtg/mumu
Knowledge bases
- KBSF Knowledge base of popular music extracted from a corpus of ∼32k documents with stories about songs. http://mtg.upf.edu/download/datasets/kbsf
- FlaBase Knowledge base of flamenco music, created by combining data from 7 different data sources, and enriched with information extracted from ∼1k artist biographies. http://mtg.upf.edu/download/datasets/flabase
Software
- ELVIS System that integrates different entity linking tools, enriching their output and providing high confident entity disambiguations. https://github.com/sergiooramas/elvis
- TARTARUS System to perform and evaluate deep learning experiments on classification and recommendation from different data modalities and their combination. https://github.com/sergiooramas/tartarus
- MEL API and demo website for a Music Entity Linking system that disambiguate musical entities to MusicBrainz. http://mel.mtg.upf.edu
Journal Publications
- Oramas S., Espinosa-Anke L., Sordo M., Saggion H. & Serra X. (2016). Information Extraction for Knowledge Base Construction in the Music Domain. Data & Knowledge Engineering, Volume 106, Pages 70-83.
- Oramas S., Ostuni V. C., Di Noia T., Serra, X., & Di Sciascio E. (2016). Music and Sound Recommendation with Knowledge Graphs. ACM Transactions on Intelligent Systems and Technology, Volume 8, Issue 2, Article 21.
- Oramas S., & Sordo M. (2016). Knowledge is Out There: A New Step in the Evolution of Music Digital Libraries. Fontes Artis Musicae, Vol 63, no. 4.
Peer-reviewed Conference Papers
- Oramas, S., Nieto O., Barbieri F., & Serra X. (2017). Multi-label Music Genre Classification from Audio, Text and Images Using Deep Features. ISMIR 2017.
- Oramas, S., Nieto O., Sordo M., & Serra X. (2017). A Deep Multimodal Approach for Cold-start Music Recommendation. DLRS-RecSys 2017.
- Espinosa-Anke, L., Oramas S., Saggion H., & Serra X. (2017). ELMDist: A vector space model with words and MusicBrainz entities. ESWC 2017.
- Oramas S., Espinosa-Anke L., Lawlor A., Serra X., & Saggion H. (2016). Exploring Music Reviews for Music Genre Classification and Evolutionary Studies. ISMIR 2016.
- Oramas S., Espinosa-Anke L., Sordo M., Saggion H., & Serra X. (2016). ELMD: An Automatically Generated Entity Linking Gold Standard in the Music Domain. LREC 2016.
- Espinosa-Anke, L., Oramas S., Camacho-Collados J., & Saggion H. (2016). Finding and Expanding Hypernymic Relations in the Music Domain. CCIA 2016.
- Oramas S., Sordo M., Espinosa-Anke L., & Serra X. (2015). A Semantic-based approach for Artist Similarity. ISMIR 2015.
- Oramas S., Gómez F., Gómez E., & Mora J. (2015). FlaBase: Towards the creation of a Flamenco Music Knowledge Base. ISMIR 2015.
- Ostuni V. C., Oramas S., Di Noia T., Serra, X., & Di Sciascio E. (2015). A Semantic Hybrid Approach for Sound Recommendation. WWW 2015.
- Oramas S., Sordo M., & Espinosa-Anke L. (2015). A Rule-based Approach to Extracting Relations from Music Tidbits. KET-WWW 2015.
- Sordo, M., Oramas S., & Espinosa-Anke L. (2015). Extracting Relations from Unstructured Text Sources for Music Recommendation. NLDB 2015.
- Oramas S., Sordo M., & Serra X. (2014). Automatic Creation of Knowledge Graphs from Digital Musical Document Libraries. CIM 2014.
- Oramas S. (2014). Harvesting and Structuring Social Data in Music Information Retrieval. ESWC 2014.
Font, F., Oramas, S., Fazekas, G., & Serra, X. (2014). Extending Tagging Ontologies with Domain Specific Knowledge. ISWC 2014.
Other conference presentations
- Oramas, S. (2017). Knowledge Extraction and Feature Learning for Music Recommendation in the Long Tail. 5th Large Scale Recommendation Systems Workshop, co-located with RecSys 2017, Como, Italy.
- Oramas, S. (2017). Discovering Similarities and Relevance Ranking of Renaissance Composers. The 63rd Annual Meeting of the Renaissance Society of America (RSA), Chicago.
- Oramas S. (2015). Information Extraction for the Music Domain. The 2nd International Workshop on Human History Project: Natural Language Processing and Big Data, CIRMMT, Montreal.
- Oramas, S., & Sordo M. (2015). Knowledge Acquisition from Music Digital Libraries. The International Association of Music Libraries and International Musicological Society Conference (IAML/IMS 2015), New York.
Tutorials and Challenges
- Oramas S., Espinosa-Anke L., Zhang S., Saggion H., & Serra X. (2016). Natural Language Processing for Music Information Retrieval. ISMIR 2016.
- Speck R., Röder M., Oramas S., Espinosa-Anke L., & Ngomo A. C. N. (2017). Open Knowledge Extraction Challenge 2017. ESWC 2017.